Data-Driven Decisions: From Siloed Mess to Strategic Success
- Tulio Rizzi
- Dec 22, 2023
- 6 min read
Updated: Jul 25, 2024
Master Data Governance & Visualization to Win Insights and Outsmart the Competition
Tulio Rizzi - IXNOVEM Editor / December,2023
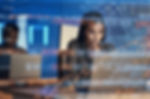
In a previous article (The End of Third-Party Cookies and How it Impacts Personalization) we discussed about the challenges companies will be facing when Google phase out third part cookies, it basically listed some other actions can be taken to keep gathering user data, here in this text we give a step forward and touch the challenges to manage that data.
It’s undoubtable the Companies growing dependence on Data to make informed decisions, and here is where the concept of Data-driven decision-making (DDDM (1)or 3DM) comes in. 3DM is the process of using data to identify trends, patterns, and insights to support better business decisions and it’s funded in 3 pillars: Data Governance, Data Analytics and Data Visualization.
What is Data Governance?
Data governance is the process of managing and overseeing the availability, usability, integrity, and security of an organization's data. It encompasses everything from data collection/storage to data access/usage.
Companies usually have several sources from where data can be collected, and we can basically divide them in 2 types: Internal and External:
Internal sources: Sales and CRM data, website registration and navigation data
External sources: Market research reports, industry trends, and consumer behavior data.
The challenge is how to combine the data and make it accessible (2)
To avoid silos of information, the data must go through a process of validation and consolidation. For example: a person may have registered in your website, but also have been added to you CRM by your sales team. The challenge is making them visible as a single ID, identifying that these 2 pieces of data belongs to the same person, the value comes from combining them in a way that one enriches the other and are accessible. This is the question Data Governance addresses
The market for Data Governance tools is highly competitive, with numerous vendors offering solutions that addresses various aspects of data governance (3), including data validation, profiling, and reconciliation. For example: IBM InfoSphere Information Governance (IIG) can connect to a wide range of data sources, including relational databases, NoSQL databases, and cloud-based data sources. Once connected, IIG can extract, transform, and load (ETL) data into a central repository, where it can be cleansed, enriched, and standardized. It’s been adopted by companies as Bank of America, Johns Hopkins Medicine, Toyota, GM, Siemens AG, Walmart, and Amazon.
Pharmaceutical companies can use IIG to consolidate data from its clinical trials database, its patient data warehouse, and its real-world data repository, allowing the company to gain a holistic view of its clinical research data
Here is a Gartner review page with some additional information about IBM InfoSphere:
Data Analytics and Data Visualization
Once the consolidation of data is done, the next step to put in place is a Data Analytics strategy to make the data useful and meaningful for the Organization. In the article “Catch them if you can: How leaders in data and analytics have pulled ahead”, McKinsey has identified that 47% of respondents say that data and analytics have significantly changed the nature of competition in their industries, when competitors started to extract novel insights from data that were traditionally unrelated or sitting in different systems, which allowed them to gain material edge by using data and analytics to improve core business
Tools such as Microsoft Power BI, Tableau and others can help companies cross the bridge between the data stored and the data insight.
MS Power BI and Tableau can be integrated, for example, to IBM InfoSphere Information Governance (IIG) to enhance data analysis and visualization capabilities. This integration enables seamless data exchange between the two platforms, allowing users to leverage IIG's data governance features within Power BI's data analysis and visualization environment, providing:
Streamlined Data Access: Users can directly access and utilize data governed by IIG, for example, within the Analytics tool (e.g.: Power BI, Tableau, etc.), eliminating the need for data duplication or manual data transfers.
Enhanced Data Quality: Users can benefit from the data quality and consistency checks, ensuring they are working with accurate and reliable data for analysis.
Simplified Data Governance Compliance: The data governance tool policies and access controls are extended into the Analytics tool, ensuring that data access and usage comply with organizational policies.
Unified Data Governance and Visualization: Users can manage and govern data within IIG while seamlessly visualizing and analyzing it in Power BI or Tableau, creating a unified data management experience.
Improved Data-Driven Decision-Making: The integration facilitates data-driven decision-making by providing users with a holistic view of data, from governance to visualization.
People & Data
While Data Governance and Data Analytics are crucial technology components for unlocking data value, effectiveness need a strong team with data and statistical expertise. This expertise goes beyond mastering tools like IBM InfoSphere, MS Power BI, or Tableau. It's about understanding data and crafting clear, accurate visualizations that guide insightful decisions.
Imagine you want to compare sales growth across regions. Choosing the wrong chart type, like a pie chart, could mask meaningful differences and obscure critical trends. Instead, a bar chart or line graph would reveal which regions are truly outperforming, enabling data-driven strategies for boosting growth.
By building a team with sharp data acumen and visualization skills, you can ensure your organization derives actionable insights from its data and navigates the right path to success.
Here is an illustrative video from "Statistics how to" with some real-life misleading charts:
How to avoid misleading data:
Establishing a standard model for visual data models defining consistent guidelines and conventions for representing and communicating data insights using visual elements. Here's a list of key items to consider:
Purpose and Audience: Clearly define the purpose of the visual data model and the intended audience. This will guide the choice of visual elements, the level of detail, and the overall design approach.
Visual Encoding: Choose appropriate visual elements, such as graphs, charts, maps, or diagrams, to effectively encode the data's characteristics and relationships. Consider the suitability of each visual element for the type of data, the desired message, and the audience's understanding and maintain visual consistency throughout the data model to ensure a cohesive and recognizable style.
Visual Hierarchy and Emphasis: Establish a clear visual hierarchy to guide the viewer's attention towards the most important data elements. Use visual cues like size, color, and position to differentiate key data points and relationships.
Color Palette and Coding: Carefully select a color palette that is accessible, consistent, and meaningful for the data being represented. Consider colorblindness and cultural associations when choosing colors.
Annotations and Labeling: Provide clear and concise annotations and labels to explain the meaning of the data, the axes, and any non-standard visual elements. Use consistent terminology and avoid jargon.
Feedback and Iteration: Gather feedback from diverse users to assess the effectiveness of the visual data model. Iterate on the design based on feedback to improve clarity, usability, and impact.
To finish, if combine all those pieces of technology, data and people together wouldn’t be a huge challenge already, think that structure is good, but too much structure could block the vision from getting new insights, so don’t become overly rigid in using these models.
Understand that there are trade-offs between standardization and innovation.
Standard models can promote consistency and efficiency, while innovation can lead to new insights and novel communication approaches.
![]() | is IXNOVEM Editor. Marketing, Sales and eBusiness Global Project & Program Mananer, specialized in FMCG industries |
FAQ
(1) What is Data-driven decision-making (DDDM or 3DM)?
Data-driven decision-making (DDDM or 3DM) is the process of using data to identify trends, patterns, and insights to support better business decisions. It is a three-pillar approach that includes:
· Data Governance: Managing and overseeing the availability, usability, integrity, and security of na Organization's data.
· Data Analytics: Using data to extract meaningful insights.
· Data Visualization: Communicating data insights concisely.
(2) What is the challenge of combining data from different sources?
Data silos are a common problem in organizations. This is when data is stored in separate systems that are not easily accessible or shareable. This can make it difficult to get a holistic view of the data and to identify trends and patterns.
(3) How can data governance tools help to overcome this challenge?
Data governance tools can help to connect data from different sources, cleanse and standardize the data, and implement access control policies. This can help to create a single source of truth for data and make it easier to share and analyze the data.
(4) What is the role of data analytics in DDDM?
Data analytics is the process of using data to extract meaningful insights. These insights can be used to improve business processes, make better marketing decisions, and increase sales.
(5) What is the role of data visualization in DDDM?
Data visualization is the process of communicating data insights concisely. This can be done using charts, graphs, maps, and diagrams. Effective data visualization can help to make data more understandable and actionable.
(6) What are the key considerations for avoiding misleading data visualizations?
There are several key considerations for avoiding misleading data visualizations. These include:
· Clearly defining the purpose and audience of the visualization
· Choosing the appropriate visual encoding for the data
· Establishing a clear visual hierarchy
· Selecting a color palette that is accessible, consistent, and meaningful.
· Providing clear and concise annotations and labels
· Gathering feedback from diverse users
(7) What is the trade-off between standardization and innovation in data visualization?
Standardized data visualization models can promote consistency and efficiency, while innovation can lead to new insights and novel communication approaches. It is important to find a balance between the two that meets the specific needs of the organization.